AI-Powered Cost Savings: The Two-Step Strategy for Payers
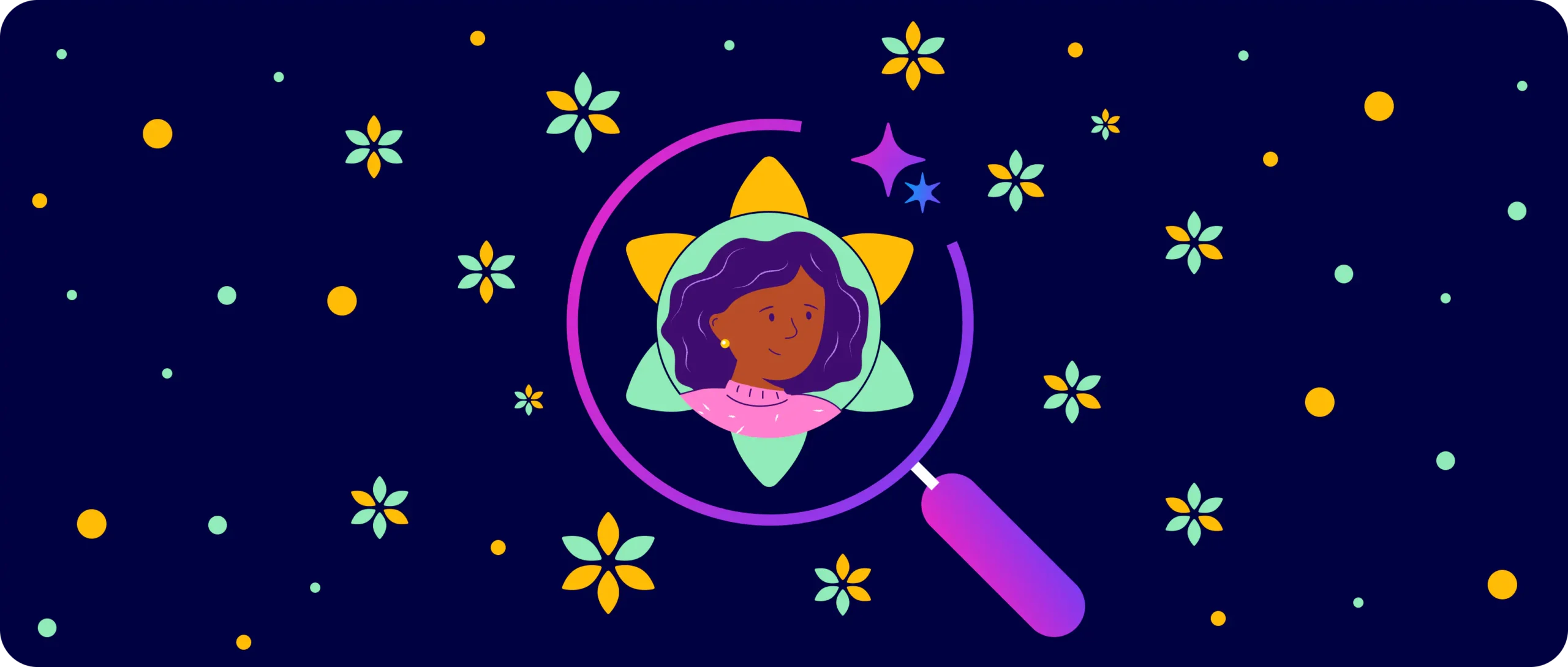
"Artificial intelligence and automation present untapped opportunity for payers...The opportunity to improve affordability, quality, and patient experience is substantial."
— McKinsey
A recently released McKinsey article delves into the transformative impact AI and automation could have on various functions within a payer organization. Despite the massive potential, many payers have yet to fully capitalize on these opportunities.
Focusing specifically on care management, we explore McKinsey's insights and the substantial cost savings AI implementation could bring specifically in the areas of administrative and medical cost reductions.
Administrative and Medical Cost Reductions:
"AI and automation could lower administrative and medical costs and increase revenue. But payers have yet to mobilize to transform their organizations to capture this opportunity."
—McKinsey
McKinsey's data suggests that integrating AI could reduce a payer’s administrative costs by $150 million to $300 million and medical costs by $380 million to $970 million for every $10 billion in revenue. Within care management, AI could account for 1.4-1.7% of administrative cost reductions and 1.7-3.6% of medical cost reductions. This translates to potential savings of $2.1 million to $5.1 million in administrative costs and $6.5 million to $35 million in medical costs for every $10 billion of revenue.
These ranges are quite large, to be sure, but they illustrate the fact that it is not just the AI technology that plays a role in successful cost reductions, but more importantly it’s application and implementation into the business’ workflows. If done correctly, AI has the potential to transform care management, which is often inefficient and inconsistent and dramatically increase the ROI from these programs.

To implement AI successfully, an organization must decide on 2 key factors about the way AI will be utilized:
- Step 1: Identification (How will your AI identify members at higher risk?)
-
- Step 2: Intervention (What steps will you take once high-risk individuals are identified?)
Key Strategies for Successful AI Implementation:
Step 1: Identification
To properly identify high-risk members, payers need to incorporate a wide range of data, including medical records, social determinants of health (SDOH), and behavioral data. AI technologies such as natural language processing (NLP) and ambient listening can gather and interpret this information, identifying the most relevant details. With advanced solutions like Laguna’s AI, care managers are even prompted to ask additional questions when potential barriers are detected, expanding the dataset further.
For example, if a care manager speaks with a diabetic single mother recovering from surgery who mentions car trouble and lack of childcare affecting her ability to attend appointments, AI can recognize this as a health barrier and flag her as high-risk.
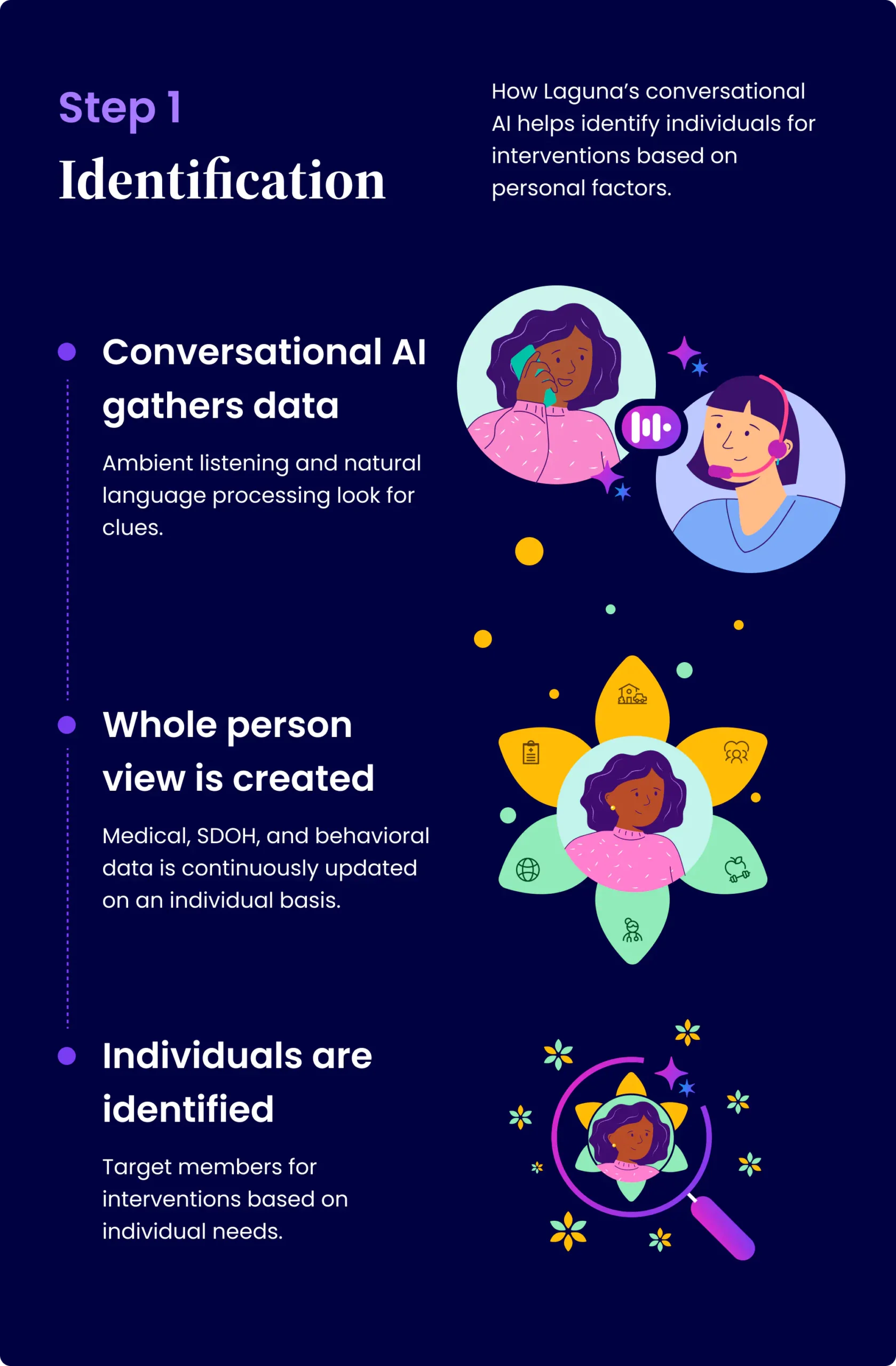
Step 2: Intervention
Intervention success often depends on the capabilities of the social worker involved, leading to variability in program outcomes. However, AI can standardize and automate interventions based on identified barriers. By learning from care manager interactions and AI-detected issues, payers can create consistent, effective programs.
For instance, AI could automatically provide childcare support for single mothers recovering from surgery or streamline the approval process for home care, ensuring reliable and uniform intervention strategies.
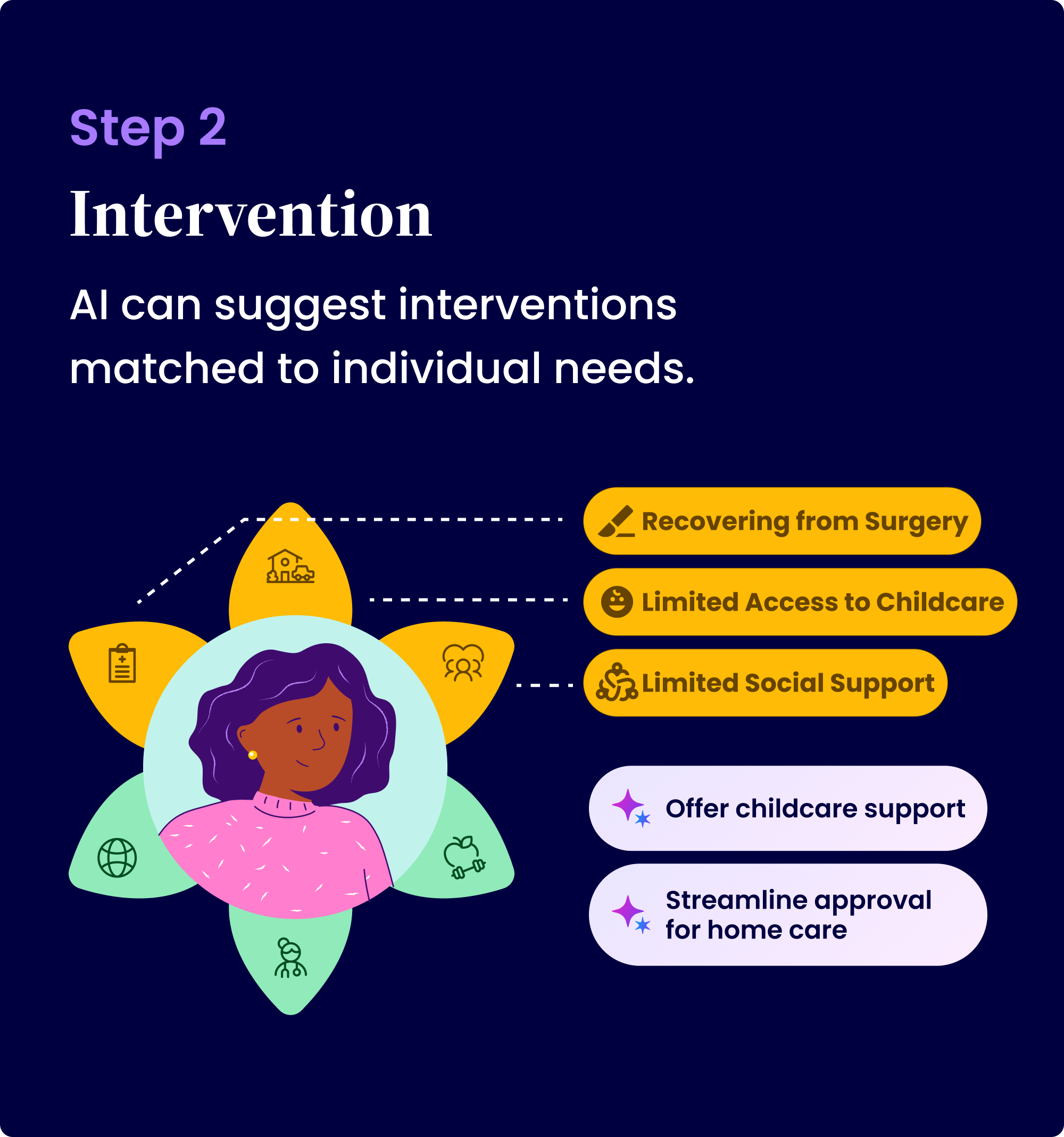
The Future of AI for Payers:
While McKinsey highlights the substantial savings AI can bring, it’s essential to recognize the even greater potential when incorporating comprehensive data and machine learning for ongoing improvements. AI in care management can lead to significant cost reductions, enhanced revenue, and market differentiation.
It’s important to note that another aspect to successful AI implementation within an organization not mentioned in this report is the collaborative and transparent approach to adoption within any business function such as care management.
At Laguna Health, we view this McKinsey study as an invitation to dive deeper into the possibilities! With conversational AI like Laguna Health, payers can go far beyond traditional inputs such as clinical information by incorporating social and emotional variables into AI models, resulting in a more in-depth dataset. This approach not only improves identification but also ensures more consistent and effective interventions.
Get in Touch 👋
Let's discuss how Laguna can help with your goals for this year.
Get in Touch 👋
Let’s talk about how Laguna can Elevate Your Care 💜 Team